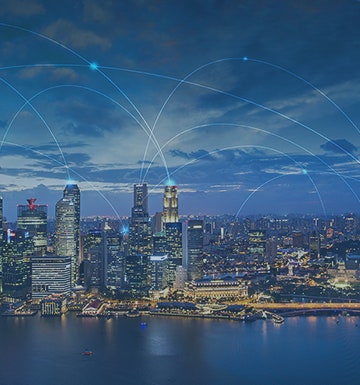
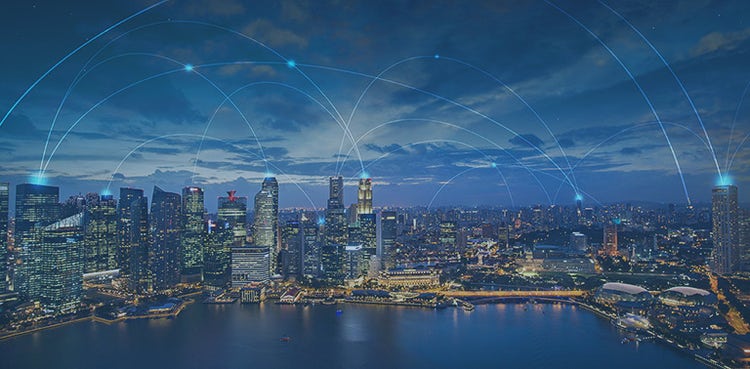
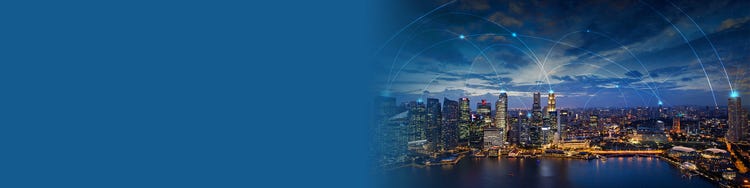
Catalysing AI tools and talent.
AI Singapore accelerates deep learning models with Adobe PDF Extract API.

40%
Reduction in number of sprints needed to deliver deployable machine learning (ML) model
Objectives
Improve quality of data ingested by natural language processing (NLP) classification pipeline
Provide only relevant content needed for corporate sustainability reporting objectives
Develop minimum viable machine learning model within 10 sprints and seven months
Results
Extracted PDF files with high accuracy to prove concept for 500 disparate documents
Implemented PDF Extract API in just two weeks
Provided data with better context and structure for superior model results
Delivered deployable model 40% faster than planned
When companies in Singapore face a tough challenge and want to explore using artificial intelligence (AI) to achieve their organisation’s goals, they turn to AI Singapore (AISG). Launched to catalyse Singapore’s capabilities to power its future digital economy with AI, the national programme brings together all Singapore-based research institutions and the vibrant ecosystem of AI start-ups and companies developing AI products to perform use-inspired research, grow the knowledge, create the tools, and develop the talent to power Singapore’s AI efforts.
Siavash Sakhavi is Assistant Head of the flagship 100 Experiments (100E) programme, which helps solve organisations’ artificial intelligence (AI) problem statements and assists them with building their own AI teams. An organisation may propose 100E problem statements where no commercial off-the-shelf (COTS) AI solution exists, which can potentially be solved by Singapore’s ecosystem of researchers and AI Singapore’s engineering team within 9 to 18 months.
Sakhavi’s team was challenged recently in a corporate sustainability reporting project with a large, multinational financial services client. The client had difficulties extracting text from disparate reports and brochures from various sources in the form of PDF documents. The project team, consisting of several AI, data, and platform engineers, plus AISG apprentices, wanted to feed the extracted information into a natural language processing (NLP) classification pipeline. However, the project team noticed the pipeline was not performing as expected because of large volumes of unstructured, gibberish text being returned by the PDF extractor tools they were using.
For typical projects, the team is able to develop models within one to two months. In this situation, it was already on sprint six of ten, so pressure was building to produce results. Fortunately, they heard about Adobe PDF Extract API, which at the time was emerging from beta to general availability. A new web service from Adobe, PDF Extract API, parses data and context from native and scanned PDF files, extracting text, table, and image elements within a structured JSON file.
“Adobe PDF Extract API was a lifesaver. Without it, we could not have used such disparate inputs to make our designed NLP solution in time.”
Siavash Sakhavi
Assistant Head, 100E, AI Singapore
Shifting gears with better structure and content extraction
“One demo later, we decided to completely switch to Adobe PDF Extract API, because it looked extremely promising,” says Sakhavi. By the end of the sprint, the team was making great progress. It was soon able to rapidly scale ingestion to its NLP pipeline, ultimately delivering the promised work to the project sponsor ahead of schedule.
“Adobe PDF Extract API was a lifesaver. Without it, we could not have used such disparate inputs to make our designed NLP solution in time,” Sakhavi says. “Up to that point, we had been building and refining how the machine learning model should work. But the other PDF extractors were giving us terrible results, creating a bottleneck in generating quality text inputs for our model.”
The open source extraction tools the team had been testing were unable to accurately identify paragraphs. Many of the sentences delivered were cut off or otherwise unusable and had to be thrown out. Others were missed entirely. Many times, numbers and labels from charts were improperly extracted as body text. The outputs lacked structure entirely.
“It’s not an easy task to go in manually and extract what you want from these texts and eventually classify them. We had to go out and find a better PDF extractor to provide automation and efficiency for our work,” says Sakhavi. “Thankfully we were able to plug in PDF Extract API just in time, right when we needed to start quickly ramping up our NLP processing volume.”
PDF Extract API delivered outputs based on paragraphs instead of just sentences and fragments. “The context data and capabilities with grouping paragraphs proved tremendously valuable. This really boosted our ingestion pipeline and drove better outcomes in our machine learning algorithm as well,” Sakhavi says.
Increasing relevancy for superior model results
“Our results were amazing,” Sakhavi says. “PDF Extract API plugged in very nicely. We made an adaptor for it to only extract exactly what we needed and ultimately the API was impressively accurate, enabling a quick acceleration against our project schedule.”
The AISG team planned to develop a Bidirectional Encoder Representations from Transformers (BERT) deep learning model. To feed the model, the project sponsor provided a keyword glossary definition for factors, each belonging to a certain label, that were important to a particular corporate Environmental, Social, and Governance (ESG) initiative. The AISG team’s goal was to perform a similarity matching process between those definitions and the documents being analysed, to determine which parts of the text were relevant for the project.
“The context for these texts was really domain-specific related to environmental sustainability, so we couldn’t train a model until we had extracted relevant text to match with the glossary information,” says Sakhavi. “PDF Extract API was able to correctly identify important sentence-level information in the context of paragraphs about topics related to sustainability, providing a high quality of data for ingestion.”
“Our results were amazing. PDF Extract API plugged in very nicely. We made an adaptor for it to only extract exactly what we needed and ultimately the API was impressively accurate, enabling a quick acceleration against our project schedule.”
Siavash Sakhavi
Assistant Head, 100E, AI Singapore
The corporate sponsor wanted to analyse 500 disparate documents from different sources, looking to Sakhavi’s team to take care of the first 10 while proving the concept, before handing over a full-fledged, deployable model that would allow the company to handle the remaining 490. “The reports and brochures involved were not straightforward by any means. There were a lot of images, in addition to text elements floating around on different parts of pages. The sizes and content arrangements had a lot of variability,” he says.
Sakhavi and his team were able to implement PDF Extract API in just two weeks, for a massive improvement in data quality. “It was a very short learning curve,” he says. The team quickly learned how to incorporate lists of data into its pipeline, parsing everything based on path attributes to achieve the desired results.
“At the end of sprint six we were able to present promising results to our corporate client turning the corner to enable a production-worthy solution with a bit more work from our team,” Sakhavi says.
Fostering an innovative Singaporean AI ecosystem
The project scope has since been expanded for research probing in a new area. It’s been handed over to a university in Singapore, working in tandem with the AI Engineering team of AISG. The good news for the corporate sponsor is that the same people who worked on the AISG project team joined the university research team to continue helping bring this initiative to fruition, ultimately training a new model using the labeled data and producing superior results.
“AISG continues to have many projects that require PDF parsing.” Sakhavi says. “My recommendation to all teams is to utilise PDF Extract API and also suggest it to the sponsoring clients for their ongoing use.”