A marketer’s guide to descriptive, predictive, diagnostic, and prescriptive analytics.
02-24-2025
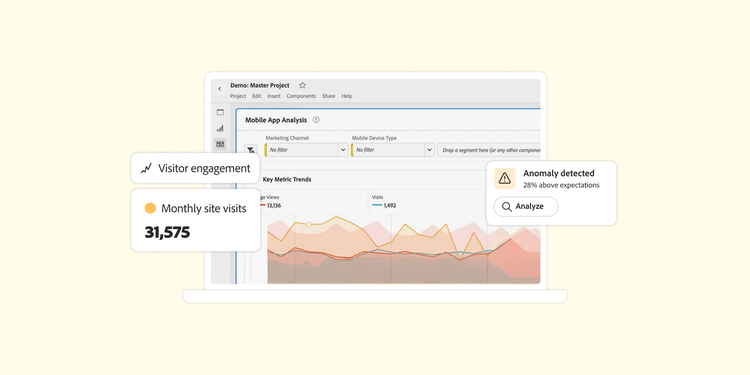
How do successful businesses turn raw data into strategic gold? The secret lies in understanding the most common types of analytics — descriptive, predictive, diagnostic, and prescriptive. In this post, you’ll learn how each type works, their benefits, and how they combine to power data-driven decisions.
In this guide:
- Types of business analytics
- What are descriptive analytics?
- What are predictive analytics?
- What are prescriptive analytics?
- How does prescriptive analytics differ from predictive and descriptive analytics?
- Diagnostic analytics
- Get started with business analytics
Types of business analytics.
Business analytics is a critical tool for interpreting the vast amount of data your company collects. From customer behavior and conversion rates to revenue trends and operational efficiency, analytics helps transform raw data into actionable insights.
The four primary types of analytics are:
1. Descriptive analytics: Understand what happened.
Descriptive analytics summarizes historical data to reveal patterns and trends, offering insights into past performance.
Example: A retailer analyzing monthly sales data identifies a spike in winter clothing sales during December, helping them prepare for seasonal demand in the future.
2. Predictive analytics: Anticipate what might happen.
Predictive analytics uses historical data, statistical models, and machine learning to forecast potential outcomes.
Example: An ecommerce platform analyzes browsing history and past purchases to recommend products customers are likely to buy.
3. Prescriptive analytics: Determine what actions to take for optimal outcomes.
Prescriptive analytics builds on predictive insights to recommend specific actions that maximize desired results.
Example: A logistics company optimizes delivery routes by factoring in historical traffic patterns, weather data, and delivery priorities, reducing costs and improving efficiency.
4. Diagnostic analytics: Explain why something happened.
Diagnostic analytics digs deeper into past data to identify the reasons behind specific trends or events.
Example: An online business experiencing a drop in sales uses diagnostic analytics to uncover that a recent website update slowed down page load times, leading to higher cart abandonment rates.
By understanding the distinctions between these analytics types, businesses can apply the right methods to support their objectives.
Descriptive analytics.
Descriptive analytics focuses on summarizing data to reveal patterns and trends. It helps businesses understand what has happened in the past or is currently happening.
Key takeaways:
- Descriptive analytics summarizes the health of a business.
- It underpins predictive and prescriptive analytics.
- It relies on operational intelligence, which looks at real-time data, and business intelligence, which focuses on historical data.
Why is descriptive analytics important?
Descriptive analytics serves as the starting point for all data analysis. Before making improvements or forecasting the future, businesses need a clear understanding of their current state.
For instance, a retailer analyzing inaccurate sales data may incorrectly assume their best-performing product is seasonal, leading to overstocking and unnecessary expenses.
High-quality descriptive analytics depends on reliable data, robust methodology, and well-defined KPIs. Poor-quality descriptive data undermines all subsequent analytics processes.
What are the different categories of descriptive analytics?
The two main categories of descriptive analytics are operational intelligence and business intelligence.
- Operational intelligence deals with real-time analysis of data currently flowing in, like data from IoT devices. It’s a proactive approach, as you can take action immediately when you see the numbers come in.
- Business intelligence is reactive. It looks at past data and derives insights based on actions that have already happened.
Predictive analytics.
Predictive analytics uses historical data, statistics, and machine learning to identify patterns and forecast potential outcomes. It provides probabilities rather than certainties, offering businesses a way to anticipate trends and risks.
Examples include ecommerce platforms recommending products based on browsing history, banks assessing creditworthiness, and marketers forecasting campaign success.
Types of predictive analytics models.
- Decision trees: Simplify decisions with a visual flowchart of possible outcomes.
- Regression models: Predict outcomes based on variable relationships (for example, predicting sales based on advertising spend).
- Neural networks: Process large datasets to uncover complex patterns.
- Cluster models: Group similar data points to identify trends (for example, segmenting customers by purchasing behavior).
- Time series models: Forecast trends based on chronological data, such as seasonal sales fluctuations.
Benefits of predictive modelling.
- Enhanced security: Detect anomalies like fraud or cybersecurity threats.
- Reduced risk: Anticipate potential problems before they occur.
- Greater efficiency: Optimize resources and prevent disruptions.
- Better decision-making: Rely on data-driven insights for strategic planning.
Prescriptive analytics.
Prescriptive analytics builds on predictive analytics by recommending specific actions for achieving desired outcomes. It uses data, machine learning, and artificial intelligence (AI) to identify optimal decisions.
Examples of prescriptive analytics:
- Insurance: Calculating client risk to personalize coverage and rates.
- Product development: Using customer surveys and market research to identify must-have features.
- Healthcare: Optimizing treatment plans to reduce patient risks.
Business benefits:
Prescriptive analytics helps businesses optimize complex processes. For example, companies can use prescriptive models to set pricing strategies by factoring in product value, development costs, and marketing expenses. For example, an airline might dynamically adjust ticket prices based on demand, weather forecasts, and competitor rates to maximise revenue.
How does prescriptive analytics differ from predictive and descriptive analytics?
Diagnostic analytics.
Diagnostic analytics explains why certain trends or events occurred by uncovering causal relationships and sequences in the data. It builds on descriptive analytics to go beyond identifying what happened, focusing instead on the reasons behind specific outcomes.
Diagnostic analytics is retrospective, providing businesses with the insights needed to address past issues or replicate successes. For instance, if an ecommerce business experiences a sudden drop in conversion rates, diagnostic analytics can uncover the root cause.
Examples of diagnostic analytics.
- Why year-over-year sales increased: Diagnostic analytics might reveal that a marketing campaign targeting a specific demographic resulted in higher sales conversions.
- Reasons behind unexpected product success: A product’s surge in popularity could be linked to a favorable review from a prominent influencer.
- Customer churn during specific periods: Analysis might show that a spike in churn coincided with increased subscription pricing or service outages.
Limitations of diagnostic analytics.
While diagnostic analytics is effective for understanding past events, its backward-looking nature limits its ability to provide actionable recommendations for the future. To create forward-looking strategies, businesses must combine diagnostic insights with predictive and prescriptive analytics, which offer guidance on what might happen next and what actions to take.
By identifying underlying causes, diagnostic analytics helps businesses prevent recurring problems and enhance outcomes, forming a crucial bridge between understanding the past and planning for the future.
Get started with business analytics.
The four types of analytics — descriptive, predictive, prescriptive, and diagnostic—equip businesses with the tools needed to understand past performance, anticipate future trends, and optimize outcomes.
To start, identify your business’s key questions and choose the analytics type that aligns with your goals. Adobe Analytics provides tools to transform data into actionable insights, enabling businesses to harness the power of AI-driven analytics.
Adobe Analytics helps businesses of any size, and in any industry, turn data into business intelligence. Collect and organize data all in one place and put the power of AI to work in analytics that create meaningful, actionable insights.
Watch the video or request a free, custom demo to see how Adobe can harness the power of analytics for your brand.