ADOBE REAL-TIME CDP
AI vs. machine learning
Artificial intelligence (AI) is how machines simulate human intelligence, usually to perform advanced tasks without human intervention. Machine learning (ML) models use rule-based algorithms to learn without additional programming.
AI and ML are often used interchangeably because they’re closely interconnected, but there is a difference between them. It’s important for organizations to understand the nuances between these two technologies to invest in the right solutions.
In this article, we’ll explain what AI and machine learning are, how they differ, how they work together, and how businesses can apply them to see greater gains in productivity.
Explore the sections of this page
What is artificial intelligence?
What is machine learning (ML)
The difference between machine learning and AI
How AI and machine learning work together
Benefits of AI and machine learning
How to apply artificial intelligence and machine learning
#application-of-artificial-intelligence-and-machine-learning
The future of AI and machine learning
What is artificial intelligence?
With AI, machines perform tasks that are commonly associated with intelligent beings. Normally, “intelligence” refers to the human and non-human (animal) capability to reason. However, “artificial” means that this intelligence is generated not by an organic being but by a computer.
In practice, AI is human-made thinking power performed by machines.
While it may sound like science fiction, AI is likely already part of your daily life. Virtual assistants like Siri and Alexa use AI to learn your preferences and suggest relevant results. AI-powered chatbots also make it possible for shoppers to get personalized help 24/7.
But AI can’t generate these insights on its own. It typically requires some form of data to complete these processes. This data could be a typed message, a vocal command, or an image.
It’s also important to remember that there are several types of AI. Organizations use one or several types of AI to accomplish different tasks.
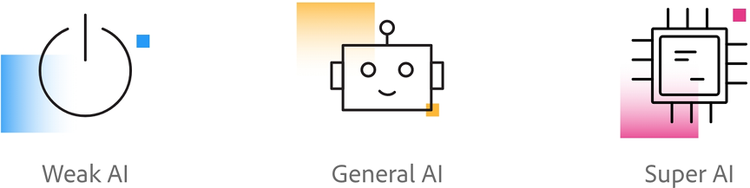
Weak AI
Weak AI is a type of AI that’s very limited, with a narrow scope of specialization. This AI can’t perform tasks beyond its defined limitations because it’s only trained for one specific task.
For example, digital voice assistants and product recommendation engines use weak AI. They can’t perform additional tasks or functions outside of their specialization.
General AI
General AI has the capacity to mimic how the human brain learns. Theoretically, General AI can perform any intellectual task with the efficiency of a human, although its capabilities are still being researched.
Super AI
Super AI is a level of intelligence at which machines surpass human intelligence. At this level, AI can perform tasks better than humans. Super AI is currently only hypothetical.
Regardless of the type of AI a business uses, this technology relies on algorithms to interpret data and simulate human-like intelligence. This is where AI diverges from machine learning, which uses a supply of pre-programmed data to perform a specific task.
Let’s look at the basics of how machine learning works.
What is machine learning (ML)?
Machine learning is a subcategory of artificial intelligence that enables machines to learn from past data or experiences. They aren’t explicitly programmed to produce algorithms for repeatedly accurate outcomes.
For example, speed-to-text search uses ML to translate spoken words into text on your phone. In the medical world, doctors use machine learning to speed up imaging analysis, and in banking, ML speeds up fraud detection.
There are three types of machine learning models.
Supervised learning
This type of ML is trained using labelled training data. Data scientists train the model using very clear, descriptive data. Once it’s trained, this type of machine learning model can classify data into categories and use that information to spot patterns and trends.
Reinforcement learning
With reinforcement learning, the ML model learns through trial and error. The model will generate an output, and it’s up to the data scientist to offer feedback on whether the output is correct or not. This allows the model to gather feedback from its past experiences to determine the correct actions to perform a task.
Unsupervised learning
Unlike supervised learning, which provides labelled data, unsupervised learning provides unlabeled datasets to an ML algorithm. This means the algorithm is able to spot patterns and groupings on its own without the need for human intervention.
ML models can be complex, but these algorithms can have a big impact on the quality and usefulness of their outputs. Learn more about how machine learning models work.
The difference between machine learning and AI
Machine learning and AI are closely related because ML is a subset of AI. However, ML has a different objective than AI, so it’s important not to mix up the two technologies.
Let’s look at the major differences between AI and machine learning.
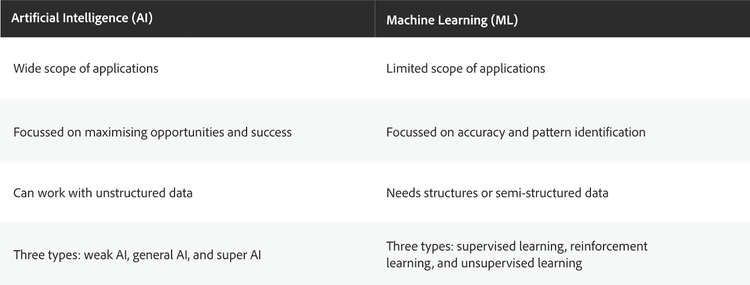
Understanding AI
With AI, data scientists create intelligent systems that can perform tasks like a human. The objective of AI is to make a smart computer system with human-like intelligence that can solve complex problems without direct human input.
AI has a wide range of applications, but it’s primarily focused on maximizing opportunities and success. Because it’s more advanced, AI can deal with unstructured data. This means data scientists can upload an unlabeled dataset and still glean strong insights.
The three types of AI are weak AI, general AI, and super AI.
Understanding machine learning
The goal of machine learning is to allow machines to learn from data so that they can produce accurate outputs. With ML models, data scientists teach machines with data as a way to perform specific tasks and produce accurate results.
ML has a limited scope of applications, and it primarily focuses on accuracy and pattern identification. It can’t make sense of unstructured data — it needs structured or semi-structured data to generate accurate outputs.
The three types of ML are supervised learning, reinforcement learning, and unsupervised learning.
How AI and machine learning work together
AI and ML work together to provide innovative solutions to real-world tasks. In fact, AI systems are built using ML.
While AI and ML are different concepts, together they produce superior results.
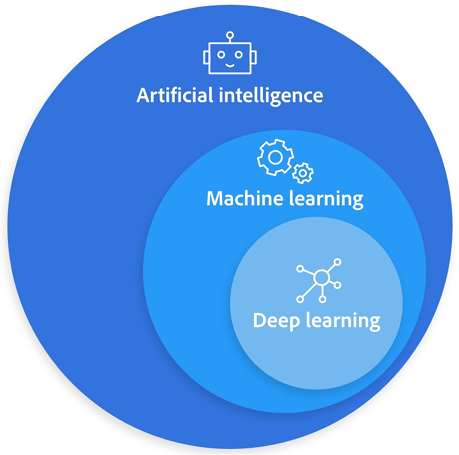
How this works
Machine learning is less complex than AI, but it’s a driving force for AI systems. AI uses ML to complete tasks such as:
- Data preparation. This involves selecting, cleaning, and transforming data into a format that can be used by an ML algorithm.
- Model training. An ML algorithm is used to build a model that can make predictions and decisions based on the input data. The algorithm “learns” from the data by identifying patterns and relationships and adjusting its parameters to minimize errors. This process is repeated several times and continued until a high level of accuracy is achieved consistently.
- Model deployment. Once the model is trained, it can be deployed in a real-world setting to make predictions or classifications based on new data as a form of artificial intelligence.
Some people assume that AI and machine learning are limited only to big companies, but these technologies are prevalent in day-to-day life. In fact, 77% of people use devices or services powered by AI — and most don’t realize it.
Deep learning is a subset of ML. It uses neural networks with three or more layers to simulate the learning abilities of the human brain. Many AI tools use deep learning to speed up automation and perform higher level tasks. Learn more about how deep learning differs from machine learning.
Benefits of AI and machine learning
AI and ML give businesses more opportunities to automate tasks and speed up their workflows. Together, AI and ML deliver benefits such as:
- More sources of data input. Businesses are awash in data. Instead of shelving this information, AI and ML allow organizations to gather and process a greater amount of data inputs. This mobilizes data at scale automatically.
- Better, faster decision-making. AI and ML allow organizations to leverage higher-quality data in large volumes. In practice, this means businesses can pull data-driven insights more regularly from unstructured data. This helps them be more agile and data-driven, which leads to improved decision-making across an enterprise.
- Increased operational efficiency. AI and ML allow businesses to automate tedious tasks while reducing errors, which makes businesses more efficient and cost-effective. In fact, AI approaches can reduce errors by as much as 50% for some industries, such as retail.
Application of artificial intelligence and machine learning
Humanity has barely scratched the surface of what AI and ML can do. However, these technologies are already growing in popularity. In fact, 35% of organizations used AI in 2022, which was a 4% increase over 2021.
In 2023, we see more businesses using artificial intelligence and machine learning in these applications:
- Customer service. Organizations are relying on AI and ML to answer customer queries more quickly — and more accurately — with AI-powered chatbots. They’re also using solutions like Adobe Real-Time Customer Data Platform (CDP) to collect deeper insights on their customers to deliver impeccable service.
- Banking and finance. Financial companies use AI and ML for everything from compliance to investment management to document processing.
- Customer insights. More businesses are able to personalize the customer experience through AI-driven insights. For example, Spotify and Amazon recommendation engines use AI to gather data and automatically deliver personalized experiences.
- Cybersecurity. IT providers are increasingly using AI and ML for detecting and mitigating security threats and preventing human errors.
- Transportation. Self-driving cars and cargo ships are just a few examples of how AI and ML streamline transportation.
- Marketing strategy. Marketers use solutions like Adobe Experience Platform to generate AI-driven, real-time customer intelligence to enhance personalization.
- Healthcare. AI and ML help healthcare providers improve diagnostic accuracy, offer personalized care via virtual assistants, and detect fraud.
The future of AI and machine learning
Going forward, we expect to see an increase in demand for AI and ML. By 2029, the AI and ML industry will be worth more than $209 billion.
In the future, AI will revolutionize medicine and research, in addition to creating hyper-personalized consumer experiences. For example, Adobe Real-Time CDP generates insights and actionable steps to scale audiences through artificial intelligence and machine learning. Demand will also increase for STEM jobs to manage AI and ML solutions. In fact, computer and data scientist jobs are predicted to grow by 21% through 2031.
It’s clear that AI and ML will continue to revolutionize business as we know it. This technology often operates in the background, but going forward, it will likely play a more active role in both our work and our daily lives.
Are you ready for the next stage of AI evolution? See how machine learning powers Adobe Sensei and all Adobe Experience Cloud products.
Recommended articles
https://main--bacom--adobecom.hlx.page/fragments/products/cards/rtcdp